Advanced microbial modelling techniques and risk-based management applied to aseptic-UHT process
- Like
- Digg
- Del
- Tumblr
- VKontakte
- Buffer
- Love This
- Odnoklassniki
- Meneame
- Blogger
- Amazon
- Yahoo Mail
- Gmail
- AOL
- Newsvine
- HackerNews
- Evernote
- MySpace
- Mail.ru
- Viadeo
- Line
- Comments
- Yummly
- SMS
- Viber
- Telegram
- Subscribe
- Skype
- Facebook Messenger
- Kakao
- LiveJournal
- Yammer
- Edgar
- Fintel
- Mix
- Instapaper
- Copy Link
Posted: 5 March 2014 | Laure Pujol and Jeanne-Marie Membré, INRA, UMR1014 Secalim and LUNAM Université, Oniris | No comments yet
Ultra High Temperature (UHT)-type products are ambient stable products, with a long shelf life (three to six months). Since they do not require any cold chain storage and can be consumed immediately, they are consumed extensively everywhere on the globe. They are defined as commercially sterile meaning that the product “must be free of microorganisms capable of growing under normal non-refrigerated conditions of storage1.” Basically, the challenge in a UHT process is to fill a ‘sterile’ product in a ‘sterile’ container in order to achieve commercial sterility.
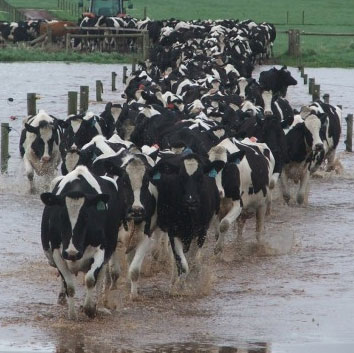
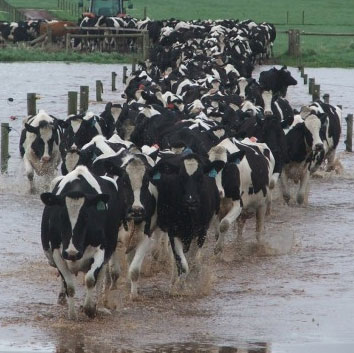
Traditionally, this complex process has been run using deterministic or empirical process settings. To move towards science-based process settings, risk of microbial contamination along the whole aseptic-UHT process must be quantified. That is now feasible due to modelling techniques dedicated to the microbial contamination variations encountered in the process, and also to the advanced probabilistic techniques enabling to take the inherent variability and uncertainty of biological processes into account. Such techniques offer the possibility of improving on the currently tolerable commercial sterility failure rate (less than one defect per 10,000 units produced). In addition, benefits of applying a risk-based management system are: i) to implement process settings in a transparent and scientific manner instead of empirically; ii) to develop a uniform common structure whatever the production line, leading to a harmonisation of these process settings, and; iii) to quantify the impact of various management measures and weigh them against their implementation cost.
The aseptic-UHT process is relatively complex but can be defined in three main phases based on the material and process flow (Figure 1, page 14)2. The first one is a ‘non-sterile’ phase, in which product ingredients and packaging raw materials are received and sterilised. A UHT treatment is applied to the product, and thermal and / or chemical sterilisation treatments are applied to the packaging. The second one is a phase where the product and packaging have to be ‘sterile’ and aseptically filled and sealed. This phase includes the most challenging steps in terms of controlling contamination risk to the product since biofilm formation, air recontamination and defective sealing can occur and cause a significant number of product sterility failures. The last phase encompasses the end product storage up to the consumer place.
Risk analysis is a systematic and comprehensive methodology to estimate the risk associated with a complex process3 and is split into three pillars: microbial risk assessment (MRA), risk management, and risk communication. For an aseptic-UHT food process with a commercial sterility target, the four steps of MRA can be defined as can be seen in Figure 12. The hazard identification consists of identifying microbiological agents capable of causing adverse health effects (for pathogens) or significant sensory deterioration of the product (for spoilage agents). The hazard characterisation is the evaluation of the nature of the adverse effect of pathogens (i.e. public health concern) and spoilage agents (i.e. spoilt product). The exposure assessment consists of the quantification of contamination along different pathways through the whole process up to consumption. Finally, the risk characterisation is the quantification of the risk in terms of public health (for pathogens) and the probability (‘risk’) to get a commercial sterility failure (for spoilage agents).
Modelling microbial variations
Mathematical models enable the quantification of microbial variations at each aseptic-UHT key process step – from raw materials reception up to end-product storage as illustrated in Figure 1. The microbial variations include reduction at the sterilisation step (for both product and packaging lines) and increase (due to growth or recontamination). In aseptic-UHT process, all contamination variations need to be considered due to the respect of the commercial sterility of the product, even if the probability of occurrence is very low.
The microbial reduction is due to the UHT treatment of the product, the sterilisation of the packaging or the cleaning procedures. The most commonly applied models in thermal treatment are the log-linear reduction, using the D and z concepts4. For packaging sterilisation, there are two processes which can be either coupled or separated5. The first one consists of heat treating the packaging with saturated steam or with a combination of super-heated steam and hot air; alternatively the packaging can be heated by extrusion using a form-fill-seal packaging system. The second process consists of applying a chemical treatment, either hydrogen peroxide or peracetic acid. Generally, the mathematical models developed to predict the probability of surviving packaging sterilisation are based on a log-linear reduction pattern2, 5. In aseptic-UHT lines, the cleaning procedures are generally split into three sequential phases: i) Cleaning-In-Place (CIP), which is an application of a chemical agent, either acid or alkaline; ii) Hot water rinsing applied to equipment surfaces; or iii) Sterilisation-In-Place (SIP), which is the re-sterilisation of the line6. A few mathematical models describe cleaning operation exits7, but their implementation in MRA models is still at an early stage.
Concerning the microbial growth, in predictive microbiology, primary models describe the log count variation over the time and secondary models the effect of storage temperature and product formulation (e.g. pH) on the lag and maximum growth rate8. In the case of growth after the heat-treatment process (e.g. storage in aseptic tank), it is sensible to consider that only one, or a few, species of microorganism have survived or have been reintroduced and have the ability to grow in the product; in such a case, microbial interactions can be neglected. A simple primary model which includes the lag (relevant response when considering thermally injured bacterial spores9) is the three phase linear model of Buchanan et al.10. A large variety of secondary models have been developed over the years8. However, for implementation in MRA models, often gamma-type models are preferred to polynomial models.
Recontamination is defined as the introduction of any microorganism into the product after an inactivation step, namely post-process recontamination. Recontamination events in aseptic-UHT processes are a primary cause of product sterility failure, and therefore processes modelling these events form a key part of any aseptic-UHT MRA model11. An overview of recontamination models has been given in Reij et al.12. Moreover, Den Aantrekker et al.13 have built a general recontamination model framework, based upon a mass-balance equation system: a contaminated source (liquid, equipment or floor) releases cells to the intermediate phase (surface, hands or air) and then cells can be transferred to the product causing recontamination.
Advanced probabilistic techniques
The aim of advanced probabilistic techniques is to deal with the variability and uncertainty inherent to biological processes14. Parameter uncertainty means uncertainty about the values of input variables, reflecting the lack of information available to estimate these values (e.g. the transfer rate parameters in the mass balance equation for recontamination). Model uncertainty also means uncertainty due to the approximation by a functional form of a real phenomenon (e.g. the recontamination by the biofilm formation). Variability differs from uncertainty: variability refers to natural or non-controlled heterogeneity between individuals within a population addressed by risk assessment (e.g. D and z values among strains of thermotolerant B. cereus). It is not always possible to separate entirely uncertainty and variability, although it is recommended to try as much as possible15. Increasingly often, probabilistic approaches (whole range of values and their probability of occurrence) are preferred to deterministic ones (single point estimate) and a large number of applications are available in the literature. However, a MRA model applied to a UHT-process line is complex; consequently it cannot be run analytically when a probabilistic approach is chosen. Therefore, numerical alternatives such as Monte Carlo simulation techniques are generally deployed. Bayesian inference is another technique used in MRA: combined to a Markov Chain technique, it enables running / solving numerically a complex model. In Bayesian inference, the natural variability is often modelled by a parametric distribution characterised by uncertain hyperparameters16.
Sensitivity analysis is widely used in MRA to: i) identify the most influential variables on the model output; ii) provide a better understanding and interpretation of the analysis; and iii) identify data gaps and then prioritise future research. Sensitivity analysis might also be seen as a prerequisite for model building, to test the robustness and the relevance of the model17. The added value of sensitivity analysis is highlighted in case of large and complex models. As mentioned by Pujol et al.2, with a MRA model applied to aseptic-UHT products, it might be difficult to identify which model modules, and inside each of these, which variables, have the greatest impact on the commercial sterility failure rate. In addition, sensitivity analysis helps in identifying and eliminating particular inputs or components of the model which are not essential, i.e. in simplifying complex models on a rational basis15.
Risk-based framework
The food safety management is now shifting from a hazard-based approach to a risk-based approach. For pathogens, the new concepts of Appropriate Level Of Protection (ALOP) and Food Safety Objective (FSO) have emerged18. FSO is defined as the maximum frequency and/or concentration of a microbiological hazard in a food at the time of consumption that provides the appropriate level of health protection. Anderson et al.19 reported the historical approaches to establishing commercial sterility and presented a perspective on the establishment of a FSO for proteolytic Clostridium botulinum19. Other examples from the literature are available in Pujol et al. 2.
On the contrary, the link between the equivalent FSO and ALOP for a spoilage hazard has not been elaborated in the literature. As pointed out by Pujol et al.2, the task of building such a ‘link’ is greatly aggravated by the almost infinite number of potential spoilage hazard agents capable of contaminating and growing within aseptic-UHT products. Nevertheless, the dose-response relationship which links ALOPs to FSOs for pathogen hazards has a counterpart in the consumer complaint and sterility failure rate targeted for spoilage hazards: a targeted sterility failure rate theoretically can be related by a food company through a measure of consumer satisfaction (e.g. consumer complaint rates).
Within the risk-based food safety management metrics, the Performance Objective (PO) is directly related to the FSO but is set at a step before the time of consumption (e.g. at the point of product release at the end of the manufacture) (Figure 2). Modelling the effect of a heat treatment step on food product post-processed contamination and making a link with the compliance to a PO or FSO has been described in the literature19-24. However, often, to be operational FSOs and POs must be translated into criteria that can be controlled and measured in the food supply chain such as Performance Criteria (PC), Process Criteria (PrC) or Product Criteria (PdC) (Figure 3). A PC is the effect required of one or more control measure(s) working in concert to meet a PO. In an aseptic-UHT process line, the air filter efficiency could be translated to a PC25. Likewise, the efficiency of the complete cleaning procedure could be set as a PC to control and reduce when necessary the contamination risk. More examples of PCs are provided in Table 1 and illustrated in Figure 3. PrC and PdC are the control parameters at a step or combination of steps that can be applied to achieve a targeted reduction or the desired limitation on growth and contamination, i.e. to achieve a PC. The type of the packaging sterilisation agent in a UHT-line could be set as a PrC. The complexity of the line (pipe length, number of valves, etc.) could be expressed in quantitative terms and then translated into PrCs; the pH of the product set as a PdC; the temperature and the time of storage set as PrCs (Table 1 and Figure 3). Once defined, PrC and PdC can be translated as Critical Control Points or Operational Pre-requisite Plans in a HACCP plan26.
Beside control measures, Microbial Criteria (MC) are commonly used in food industry. In the near future, as food safety management moves towards risk-based food management, food producers will need to provide evidence that their foods at the moment they are eaten, comply with an FSO27. For C. botulinum, an example of an FSO might be less than one product unit contaminated per 106 or 109 units19. Setting MC to assess such low occurrences is not realistic. However, MC might be valuable tools to assess the microbial quality (food spoilage) along the entire aseptic-UHT product process, i.e. to assess the compliance with POs set at various critical process steps: raw materials, mix blends pre-UHT, product units after filling, etc. (Table 1).
References
- Codex Alimentarius Commission. (1993). Recommended international code of hygienic practice for low and acidified low acid canned food. CAC/RCP 23-1979
- Pujol, L., I. Albert, N. B. Johnson, and J.-M. Membré. (2013). Potential application of quantitative microbiological risk assessment techniques to an aseptic-UHT process in the food industry. International Journal of Food Microbiology. 162:283-296.
- World Health Organisation and Food Agricultural Organisation. (1995). Application of risk analysis to food standards issues, Report of the joint FAO/WHO Expert Consultation. Available at: http://www.who.int/foodsafety/publications/micro/en/march1995.pdf. Date last accessed: 26th of November 2013
- Bean, D., F. Bourdichon, D. Bresnahan, A. Davies, A. Geeraerd, T. Jackson, J.-M. Membré, B. Pourkomailian, P. Richardson, M. Stringer, M. Uyttendaele, and M. Zwietering (2012). Risk assesment approaches to setting thermal processes in food manufacture, ILSI Europe (Ed.), 1st ed., Brussels, Belgium
- Ansari, I. A., and A. K. Datta. (2003). An overview of sterilization methods for packaging materials used in aseptic packaging systems. Food and Bioproducts Processing. 81:57-65
- Davey, K. R., S. Chandrakash, and B. K. O’Neill. (2013). A new risk analysis of Clean-In-Place milk processing. Food Control. 29:248-253
- Lelièvre, C., G. Antonini, C. Faille, and T. Bénézech. (2002). Cleaning-in-place: modelling of cleaning kinetics of pipes soiled by Bacillus spores assuming a process combining removal and deposition. Food and Bioproducts Processing. 80:305-311
- McKellar, R. C., and X. Lu (2004). Modeling microbial responses in food, R. C. McKellar and X. Lu (Ed.), 1st ed. CRC Press, New York
- Daelman, J., A. Sharma, A. Vermeulen, M. Uyttendaele, F. Devlieghere, and J.-M. Membré. (2013). Development of a time-to-detect growth model for heat-treated Bacillus cereus spores. International Journal of Food Microbiology. 165:231-240
- Buchanan, R. L., R. C. Whiting, and W. C. Damert. (1997). When is simple good enough: a comparison of the Gompertz, Baranyi, and three-phase linear models for fitting bacterial growth curves. Food Microbiology. 14:313-326
- Agalloco, J., J. Akers, and R. Madsen. (2004). Aseptic processing: a review of current industry practice. Pharmaceutical Technology. 126-150
- Reij, M. W., E. D. Den Aantrekker, and ILSI Europe Risk Analysis in Microbiology Task Force. (2004). Recontamination as a source of pathogens in processed foods. International Journal of Food Microbiology. 91:1-11
- Den Aantrekker, E. D., R. M. Boom, M. H. Zwietering, and M. van Schothorst. (2003). Quantifying recontamination through factory environments – a review. International Journal of Food Microbiology. 80:117-130
- Thompson, K. M. (2002). Variability and uncertainty meet risk management and risk communication. Risk Analysis. 22:647-654
- Mokhtari, A., and H. C. Frey. (2005). Recommended practice regarding selection of sensitivity analysis methods applied to microbial food safety process risk models. Human and Ecological Risk Assessment: An International Journal. 11:591-605
- Delignette-Muller, M. L., M. Cornu, R. Pouillot, and J. B. Denis. (2006). Use of Bayesian modelling in risk assessment: application to growth of Listeria monocytogenes and food flora in cold-smoked salmon. International Journal of Food Microbiology. 106:195-208
- Saltelli, A. (2002). Sensitivity analysis for importance assessment. Risk Analysis. 22:579-590.
- International Commission on Microbial Specification for Foods (2002). Microorganism in foods 7: Microbial testing in food safety management, ICMSF (Ed.), 1st ed. Kluwer Academic and Plenum Publishers, New York
- Anderson, N. M., J. W. Larkin, M. B. Cole, G. E. Skinner, R. C. Whiting, L. G. Gorris, A. Rodriguez, R. Buchanan, C. M. Stewart, J. H. Hanlin, L. Keener, and P. A. Hall. (2011). Food Safety Objective approach for controlling Clostridium botulinum growth and toxin production in commercially sterile foods. Journal of Food Protection. 74:1956-1989
- Membré, J.-M., (2012). Setting of thermal processes in a context of food safety objectives (FSOs) and related concepts. In: V. Valdramidis and J. F. M. Van Impe (Ed.), Progress on Quantitative Approaches of Thermal Food Processing, 1st ed. Nova Science Publishers, New York, 297-326.
- Cerf, O., and K. R. Davey. (2001). An explanation of non-sterile (leaky) milk packs in well-operated UHT plant. Food and Bioproducts Processing. 79:219-222
- Malakar, P. K., G. C. Barker, and M. W. Peck. (2011). Quantitative risk assessment for hazards that arise from non-proteolytic Clostridium botulinum in minimally processed chilled dairy-based foods. Food Microbiology. 28:321-330
- Membré, J. M., J. Bassett, and L. G. M. Gorris. (2007). Applying the Food Safety Objective and related standards to thermal inactivation of Salmonella in poultry meat. Journal of Food Protection. 70:2036-204
- Sosa Mejia, Z., R. R. Beumer, and M. H. Zwietering. (2011). Risk evaluation and management to reaching a suggsted FSO in a steam meal. Food Microbiology. 28:631-638
- Anonymous (2005). Guidelines on air quality standards for the food industry (2nd edition), K. L. Brown (Ed.), 1st ed. Campden & Chorleywood Food Research Association Group, Chipping Campden, UK
- Membré, J.-M., (2014). HAZARD APPRAISAL (HACCP) | Establishment of performance criteria. In: C. Batt and M.-L. Tortorello (Ed.), Encyclopedia of Food Microbiology, 2nd ed. Elsevier, Amsterdam
- van Schothorst, M., M. H. Zwietering, T. Ross, R. L. Buchanan, and M. B. Cole. (2009). Relating microbiological criteria to Food Safety Objectives and Performance Objectives. Food Control. 20:967-979
About the author
Jeanne-Marie Membré has a diploma in Food Engineering and a PhD in Food Microbiology and has worked in both academia and industry. In 1989, she joined the French National Institute for Agricultural Research (INRA) of Villeneuve d’Ascq, France, where she was in charge of the predictive microbiology research programme. From 2003 to 2009, she worked at the Safety & Environmental Assurance Centre of Unilever, in Bedford, UK, where she developed predictive models and exposure assessment models for a wide range of food applications. Since 2010, she has been working in INRA of Nantes, France. Jeanne-Marie is currently the leader of the Microbiological Risk Assessment in Food Group at the UMR1014 Secalim. She is involved in a large number of research projects but also in educational programme such as the Erasmus Intensive Programme Predictive Modelling and Risk Assessment. Her experience encompasses predictive microbiology, microbial risk assessment, applied statistics and food safety. Jeanne-Marie has published more than 50 articles in peer-reviewed journals, is a member of the International Association of Food Protection, belongs to the scientific board of Journal of Food Protection and is expert at ILSI-Europe.